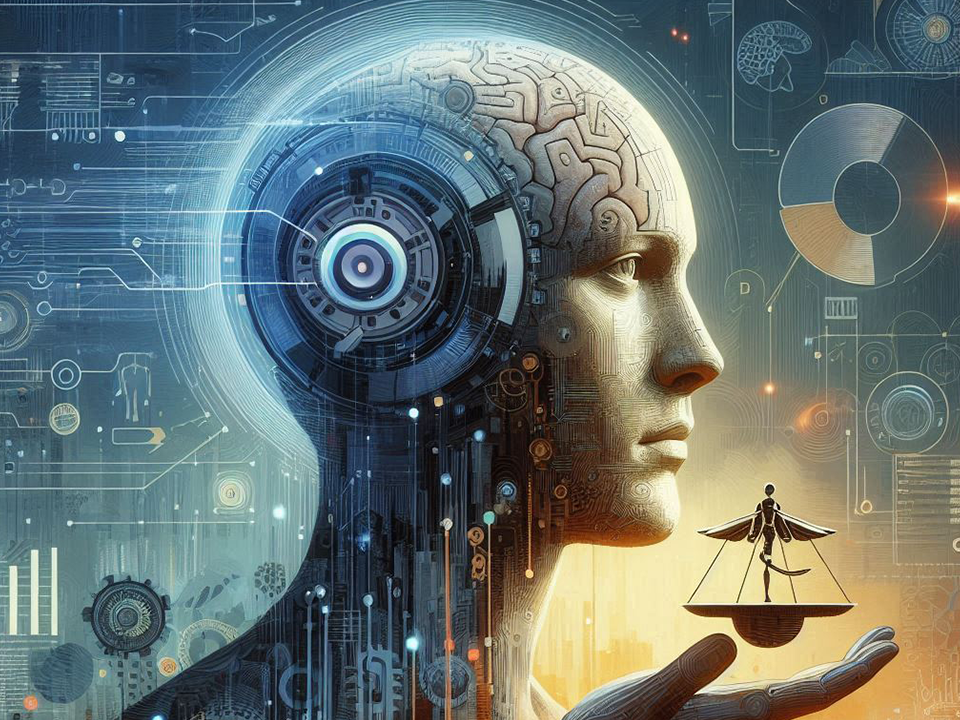
Table of Contents
First Principles of LLMs vs Machine Translations : Tapping into the Potential of AI for Efficient Localizations & Translations Workflow
With increasing awareness around AI and the improvement in the general capability of LLMs, one may have some basic questions around translation management systems using MT v/s Translation management systems powered by AI and LLMs.
This blog explores the transformative role of AI in translation, focusing on the differences between Language Learning Models (LLMs) and traditional Machine Translations, and how companies can leverage these technologies for better outcomes.
Role of Translation in Global Companies
Translations are a key factor in the success of multinational companies.
It enables businesses to reach a wider audience, engage with customers in their native languages, and foster better relationships across different markets.
High-quality translation ensures that messaging around your product and brand are accurately conveyed, reducing the risk of miscommunications and enhancing customer experience.
Introduction to Machine Translation and Language Learning Models (LLMs) and Their Differences
Machine Translation (MT) refers to the use of software to translate text from one language to another. This technology has been around for decades, evolving from rule-based systems to more sophisticated neural networks. Language Learning Models (LLMs), on the other hand, are a type of AI that can understand and generate human-like text based on vast amounts of data. While both aim to bridge language barriers, their approaches and capabilities differ significantly.
Section 1: Understanding LLMs and Machine Translations Definition and Concept of LLMs
Language Learning Models are advanced AI systems trained on extensive datasets to understand and produce human language. They leverage deep learning techniques to recognize patterns, context, and nuances in text, enabling them to generate more natural and coherent translations. LLMs like OpenAI’s GPT-4 can perform a variety of language tasks beyond translation, such as summarization, question answering, and content generation.
Definition and Understanding of Machine Translations
Machine Translation involves the automatic conversion of text from one language to another using predefined algorithms. Traditional MT systems, such as Google Translate, rely on statistical and neural methods to translate text. While they have improved over time, they often struggle with maintaining context and producing translations that feel natural to native speakers.
Key Differences Between LLMs and Machine Translations
Translation systems using Large Language Models (LLMs) and traditional Machine Translation (MT) approaches differ in several key aspects:
1. Architecture and Approach
LLMs:
- LLMs, such as GPT-4, are based on transformer architecture and are trained on vast amounts of diverse text data.
- They are not specifically designed for translation but can perform translation as one of many language tasks.
- LLMs generate translations by understanding and generating text based on the context provided.
Traditional MT Systems:
- Early MT systems used rule-based or statistical methods (like SMT – Statistical Machine Translation).
- Modern MT systems often use Neural Machine Translation (NMT), also based on the transformer architecture but specifically optimized for translation tasks.
- NMT systems are typically trained on parallel corpora (bilingual text pairs) to directly learn mappings between source and target languages.
2. Training Data
LLMs:
- Trained on a broad range of internet text, encompassing various domains, styles, and languages.
- Do not rely solely on parallel corpora but use a large and diverse dataset which includes monolingual and multilingual text.
Traditional MT Systems:
- Primarily trained on parallel corpora, which consist of text pairs in the source and target languages.
- Focus on specific language pairs and domains, often requiring curated datasets to improve performance in particular contexts.
3. Flexibility and Generalization
LLMs:
- Highly versatile, capable of performing multiple tasks beyond translation, such as summarization, question answering, and text generation.
- Can generalize across tasks due to their extensive training on diverse data.
Traditional MT Systems:
- Specialized for translation tasks, often providing high-quality translations for specific language pairs.
- May require separate models or significant retraining to handle additional tasks or languages effectively.
4. Contextual Understanding
LLMs:
- Excel at understanding and maintaining context over longer passages of text.
- Can handle nuanced language features such as idioms, colloquialisms, and cultural references due to their broad training data.
Traditional MT Systems:
- May struggle with long-range dependencies and maintaining context over lengthy texts.
- Typically perform well with more straightforward, formal language but can struggle with informal or idiomatic expressions.
5. Adaptability and Fine-tuning
LLMs:
- Can be fine-tuned on specific tasks or domains using smaller, task-specific datasets.
- Show a high degree of adaptability to new tasks with minimal additional training.
Traditional MT Systems:
- Require extensive parallel corpora for training and fine-tuning, particularly for new language pairs or specialized domains.
- Less flexible in adapting to new tasks outside of translation.
6. Performance and Applications
LLMs:
- Provide good overall performance across a wide range of languages and tasks, often outperforming traditional MT in less common language pairs or highly contextual translations.
- Used in applications where versatility and broad language understanding are crucial, such as chatbots, virtual assistants, and content generation.
Traditional MT Systems:
- Often achieve higher performance for well-supported language pairs with extensive parallel corpora.
- Commonly used in applications requiring high-precision translation, such as legal documents, technical manuals, and professional translation services.
7. Inference and Real-Time Translation
LLMs:
- Can be slower and more computationally intensive due to their large size and complexity.
- Ongoing research aims to optimize their inference speed and efficiency for practical applications.
Traditional MT Systems:
- Typically more optimized for real-time translation and can operate more efficiently in resource-constrained environments.
- Well-suited for applications requiring fast, real-time translation such as travel apps and real-time communication tools.
Section 2: How AI is Revolutionising Translations : Future Forecast & Potential
1. Improved Accuracy and Fluency
- Advanced LLMs and NMT: With ongoing improvements in Large Language Models (LLMs) and Neural Machine Translation (NMT), AI systems will become even more accurate and fluent in translating text. These models will handle complex sentence structures, idiomatic expressions, and cultural nuances more effectively.
- Contextual Awareness: Future AI translation systems will better understand and maintain context across longer texts, ensuring coherent and contextually appropriate translations.
2. Multilingual Capabilities
- Universal Translators: AI will continue to enhance its ability to translate between a broader range of languages, including low-resource and endangered languages. This will help preserve linguistic diversity and make content accessible to a wider audience.
- Code-Switching and Multilingual Texts: Advanced AI models will handle multilingual texts and code-switching (alternating between languages within a sentence or conversation) more seamlessly.
3. Real-Time Translation
- Instant Communication: Real-time translation systems, such as those used in live conversations and meetings, will become more accurate and responsive, reducing latency and improving user experience.
- Wearable Devices: Integration with wearable devices (e.g., smart glasses, earphones) will facilitate instant translation in everyday interactions, enhancing communication in multilingual environments.
4. Specialized Domain Translations
- Industry-Specific Models: AI will develop more specialized models tailored to specific industries (e.g., legal, medical, technical), ensuring higher accuracy and reliability for professional use cases.
- Terminology Management: Enhanced capabilities for managing and updating industry-specific terminology will improve the precision of translations in specialized fields.
5. Accessibility and Inclusion
- Content Localization: AI will make it easier to localize content for different regions and cultures, ensuring that information is accessible and relevant to diverse audiences.
- Assistive Technologies: AI translation will play a crucial role in assistive technologies for individuals with disabilities, such as real-time captioning and translation for the deaf and hard of hearing.
6. User-Centric Customization
- Personalized Translations: AI systems will offer more personalized translation options, adapting to individual user preferences and styles.
- Interactive Learning: Users will be able to interact with translation systems to provide feedback and corrections, allowing AI to learn and improve continuously.
7. Integration with Other Technologies
- AI and AR/VR: Integration with augmented reality (AR) and virtual reality (VR) will enable immersive translation experiences, such as real-time language overlays in AR applications.
- IoT and Smart Environments: AI translation will be embedded in smart environments and IoT devices, facilitating seamless multilingual interactions in smart homes, offices, and public spaces.
8. Ethical and Responsible AI
- Bias Mitigation: Ongoing research and development will focus on reducing biases in translation models, ensuring fair and equitable treatment of all languages and dialects.
- Data Privacy: Enhanced measures for data privacy and security will protect user information and build trust in AI translation systems.
9. Cross-Cultural Understanding
- Cultural Sensitivity: AI will improve in recognizing and respecting cultural differences, leading to translations that are not only linguistically accurate but also culturally appropriate.
- Global Collaboration: Enhanced translation capabilities will facilitate international collaboration in fields such as research, education, and business, breaking down language barriers and fostering global cooperation.
Best Practice Examples of Companies Leveraging AI in Their Translation Workflows : Ways That Companies Can Incorporate AI in Their Translation Process
- Automated Translation Tools: Integrating AI-powered tools into existing workflows to handle routine translation tasks.
- Hybrid Approach: Combining human translators with AI to ensure high-quality translations while maintaining efficiency.
- Custom Training: Training LLMs on company-specific terminology and content to improve relevance and accuracy.
- Real-Time Translation: Implementing real-time translation features in customer service and support platforms.
Case Study: Success Story of a Company That Has Adopted LLM
Consider a global tech company that invested in an AI & LLM powered translations management platform -Vaga.ai for its marketing, product documentation and customer support translations. The company was previously using an MT based platform to power its translations. Below were some of the benefits that the company experienced immediately switching to an LLM based translations platform
- No more delayed Marketing Campaigns : With Vaga.ai’s intuitive content creation, translation and reviewing capabilities, the team eliminated the external agencies and translators to review and revise merely content based translations. Thanks to LLMs contextual understanding, the tech giant was now able to work with an LLM translated content, make edits and quickly go to market with the localised content.This reduced their campaign launch time by nearly 89% which resulted in significant cost and time savings.
- 5X Content ROI & Streamlined Agency Review Workflow : Due to the streamlined process of translating the content and the capabilities of the Vaga.ai platform to translate all formats of content, the team was able to manage translating content formats across the customer journey such as blogs, youtube videos, webinars, battlecards in over 5+ languages. All this meant that the team could reuse their translated content across other forms of media – such as transcribing a podcast into multiple blog posts, subtitling English videos into several local languages and reusing some of the translated PDFs to create personalised brochures and battle cards. This also helped the team launch multi-modal campaigns across various channels leveraging various formats of the content assets.
- Consistency in content, brand & product messaging : Since Vaga.ai is also a translations workflow management tool, the teams across 5-6 different countries could quickly review and approve their content all in one platform-this helped the global team in streamlining the content approval process that resulted in consistent content and brand messaging in different content types (blog, ebooks, product demo videos, social media posts and images, landing pages etc) across various media channels.
Section 3 : Step-by-Step Guide on How Companies Can Integrate LLMs into Their Workflow
- Assessment: Evaluate current translation needs and identify areas where LLMs can add value such as translating multi-modal content assets in real time
- Selection: Choose the appropriate LLM based on the company’s specific requirements.
- Training: Train the LLM on relevant data, including a glossary on industry-specific terms and company-specific content.
- Integrations: Implement the LLM into existing translation workflows and systems such as your marketing automation tools like Marketo, Hubspot, your content management systems such as wordpress or webflow, image editing systems like Figma etc.
- Testing: Conduct thorough testing to ensure the LLM delivers accurate and contextually appropriate translations.
- Review Workflow : Establish an optimised review workflow that works for you within teams and across teams .
- Monitoring: Continuously monitor the LLM powered AI translation management platform’s performance and make adjustments in the approval and review process as needed to maintain high translation quality.
Conclusion
In summary, AI-powered LLMs offer clear advantages over traditional machine translations, including improved precision, real-time capabilities, versatility, and higher context comprehension. As businesses strive for better global reach, adopting these advanced translation technologies can provide a significant competitive edge. Embracing the future of translation with AI-powered LLM based Translation Management systems can enhance communication, streamline workflows, and connect with a global audience more effectively.